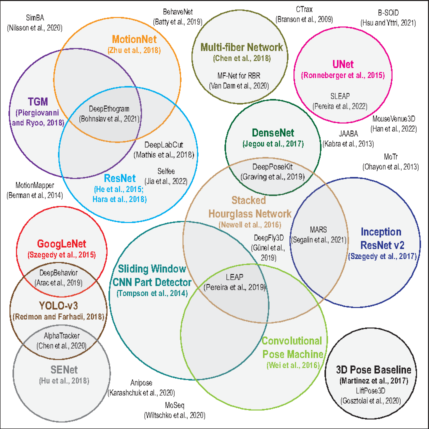
CNN-Based Action Recognition and Pose Estimation for Classifying Animal Behavior from Videos: A Survey
Perez M. and Toler-Franklin C. CNN-Based Action Recognition and Pose Estimation for Classifying Animal Behavior from Videos: A Survey. arXiv:2301.06187. 2023 January; DOI: https://doi.org/10.48550/arXiv.2301.06187
Abstract
Classifying the behavior of humans or animals from videos is important in biomedical fields for understanding brain function and response to stimuli. Action recognition, classifying activities performed by one or more subjects in a trimmed video, forms the basis of many of these techniques. Deep learning models for human action recognition have progressed significantly over the last decade. Recently, there is an increased interest in research that incorporates deep learning-based action recognition for animal behavior classification. However, human action recognition methods are more developed. This survey presents an overview of human action recognition and pose estimation methods that are based on convolutional neural network (CNN) architectures and have been adapted for animal behavior classification in neuroscience. Pose estimation, estimating joint positions from an image frame, is included because it is often applied before classifying animal behavior. First, we provide foundational information on algorithms that learn spatiotemporal features through 2D, two-stream, and 3D CNNs. We explore motivating factors that determine optimizers, loss functions and training procedures, and compare their performance on benchmark datasets. Next, we review animal behavior frameworks that use or build upon these methods, organized by the level of supervision they require. Our discussion is uniquely focused on the technical evolution of the underlying CNN models and their architectural adaptations (which we illustrate), rather than their usability in a neuroscience lab. We conclude by discussing open research problems, and possible research directions. Our survey is designed to be a resource for researchers developing fully unsupervised animal behavior classification systems of which there are only a few examples in the literature.
DOI: https://doi.org/10.48550/arXiv.2301.06187 | Download pre-print https://arxiv.org/pdf/2010.00641.pdf | BibTex Entry